In Conversation with Yelizaveta Areshka – Tech Recruiter
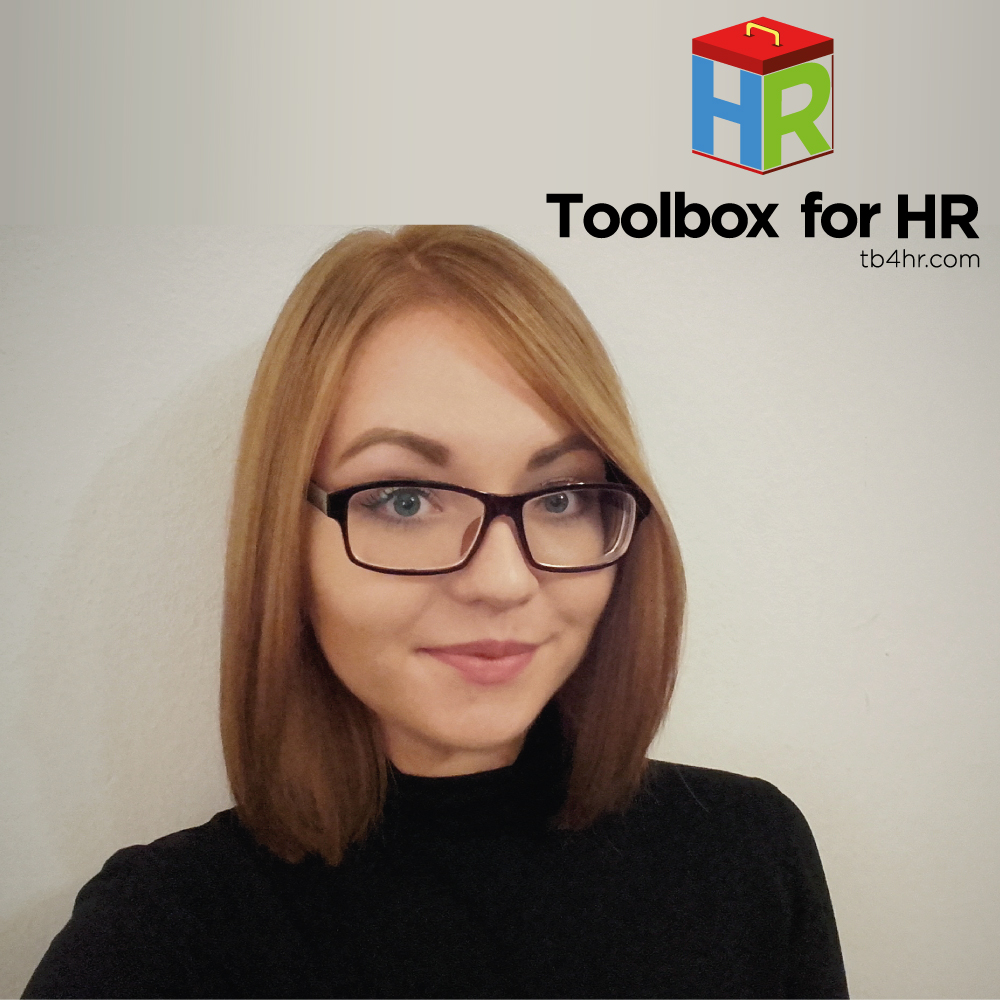
Q: You studied Business Economics. How is tech recruitment working out for you?
A: To start with, I’ve never thought that my first job ever will be connected with recruitment. My educational background already suggests that I was far from tech world before I found about TB4HR. However, after working here for half a year it became more and more obvious that understanding basic economics’ concepts helps in detecting trends and getting better understanding of why things work out this particular way, not the other. Even though both of the areas seem to be very strict to numbers and science, at the end of the day, it’s all about humans’ behaviour and decisions. Studying economics involves a lot of market analysis; so, I assume, throughout 3 years I’ve gained a decent understanding of which countries (mainly European states) have competitive advantage in terms of employment opportunities alongside with skillful labour force. Moreover, being aware of the current economic situation might allow to, sort of, “juggle” with talent pools, which is definitely beneficial for recruiters, developers and our clients. The first “wow” moment when it all perfectly matched in my head has happened when I understood that TB4HR is following the two-sided market theory in its everyday activity: we are aiming to help two sets of agents (our clients and developers) to “trade” with the help of the TB4HR platform, thus increasing their efficiency.
Q: What was the hardest thing for you when getting into recruiting? Please elaborate.
A: I believe that working as a tech recruiter has a number of its own peculiarities, when comparing to other kinds of recruitment. Hence, I had to make an extra-effort to learn basic tech notions like types of developers, programming languages, frameworks, etc. As IT developers are extremely passionate about their work (or rather a lifestyle), it definitely helps to know as much as possible about their average mindset and speak the same “language”. Without that it’s very hard to make them interested and involved into a new project, as a recruiter needs to understand the values developers have. From what I observed, it’s about the idea and challenges, not that much about the material reward.
Q: You finished thesis on immigration. What do you think about the mobility of the tech talent in Europe?
A: In my opinion, the mobility of tech talent drastically varies in different parts of Europe.
For example, there’s a common belief that developers from Eastern European countries are more prone to move to the west. It is still true in the majority of cases, however talking to the younger generation of tech talent this opinion seems to be losing its reliability. As with any concept connected to immigration, it’s very hard to evaluate a human factor: developers tend to opt for remote or freelance job instead of relocating.
If we are talking about EU states, once again, barriers of labour movement seem to be reduced to its maximum as it’s one of the 4 freedoms any EU citizen has. Nonetheless, differences in ethnical background, languages, and cultures put certain limits on the degree of mobility.
Constant changes that Europe undergoes bring gains, as the aftermath of Brexit.
For example being the largest diaspora in the UK, a lot of Polish people are moving back. It’s definitely beneficial for the labour market in Poland, as it obtains many skilled workers with extensive experience and average salary expectations, but unfortunate for the British market, as it loses skilled labour force with initially depreciated wages.
Q: What’s the future of Machine Learning? How will it impact industries and businesses?
A: Machine Learning is a big word now in numerous industries. Coming back to the field of my bachelor studies, it turned out that I’ve already encountered machine learning techniques when writing my thesis. The major goal of Machine Learning can be summed up with one word – prediction – and it is very much in line what economists and econometricians are trying to achieve by creating models. However, as innovative and as it sounds, Machine learning creates multiple challenges in the process of fitting the data: it, of course, does a great job in forecasting, but is not the best way in assessing the effects an economic policy might have on various variables.
Nonetheless, applying machine learning in the field of finance is an awesome way to predict trends and provide economic agents with solutions to simple financial problems (analysing customers’ spending patterns and suggesting saving/investment portions of their income, for example).
Q: What have you learned about tech talent while contributing #TB4HRLabs studies?
A: Having an opportunity to contribute to #TB4HRLabs studies is a great way to learn about the distribution of tech talent around the world in the quickest way possible. The first statistical research I’ve done at TB4HR was aimed on analysing tech pool in the Eastern Europe. It’s extremely helpful to be involved in these studies for a recruiter-newbie, as you can observe the density of tech pool in different countries, what programming languages are spread there, and form general opinion on which regions have the majority of certain types of developers. It always useful to give a data-backed recommendations of talent pools for our clients or provide them with alternatives.